Overview
Note: The 🔵 symbol means some students have already signed up (# indicated in brackets), but spots remain.
Capacity
We won’t be able to fill every project position. This is a rough measure of how much capacity Prof. Muise and the MuLab has remaining for capstone projects:
Application Procedure
To apply for any of the projects, please email Prof. Muise with the following details:
-
Your name and a bit of background about yourself.
-
The project name and expression of your interest in the area.
-
Your Queen’s transcript (unofficial is fine).
-
(if available) A CV/Resume
-
(if available) A link to any software/projects you’ve worked on (e.g., GitHub profile).
Over the coming weeks, we will reach out to interested students and possibly interview if there is high demand for the project. If necessary, the interview process will involve a small coding exercise as well as meeting with Prof. Muise and/or Mu Lab members.
Project Ideas
A short summary of each project is below.
Automated Agriculture Software Stack
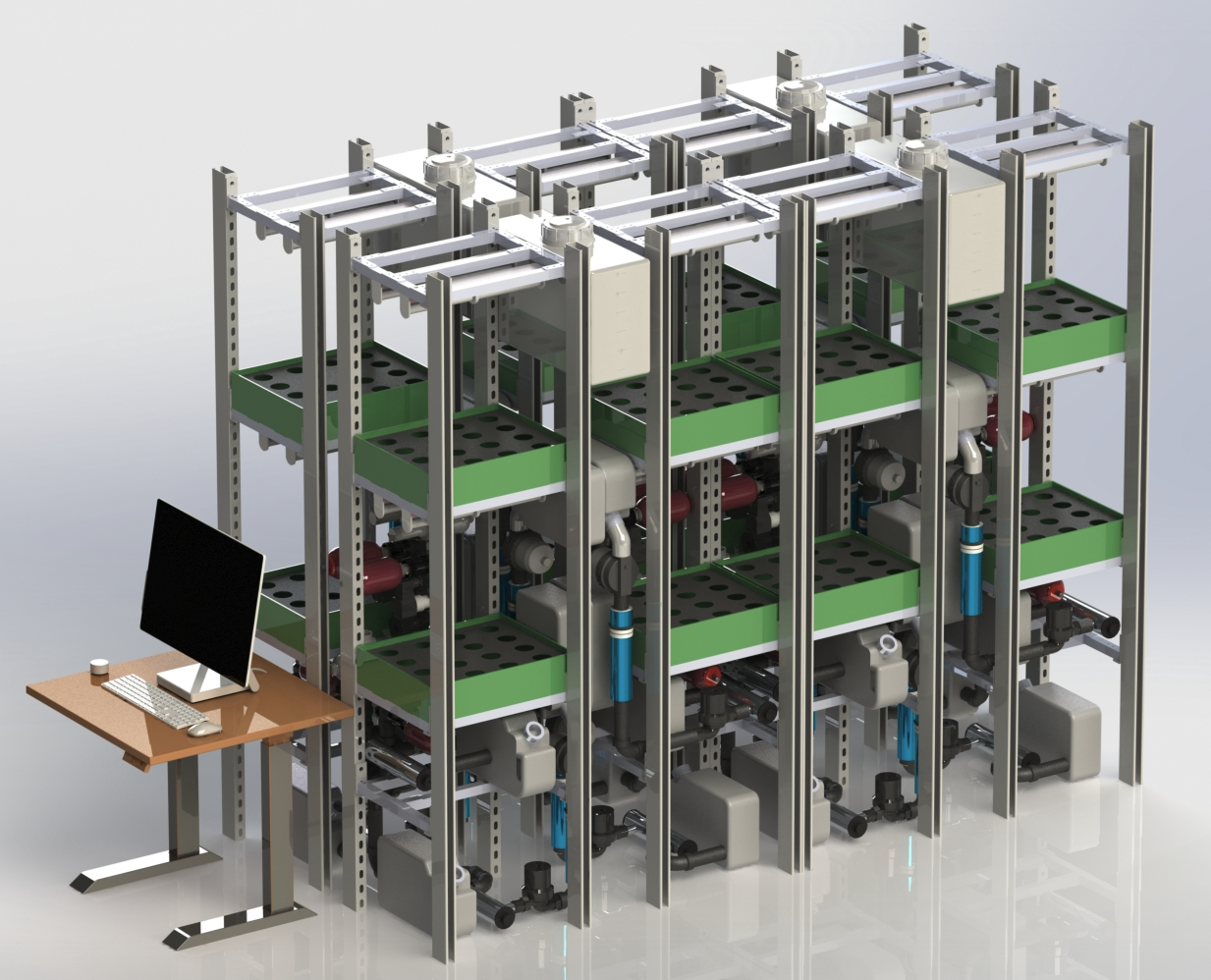
The MuLab and
Machine Intelligence & Biocomputing (MIB) Laboratory are building a lab-scale platform for the exploration of autonomous agriculture. This will include sensors such as video feeds, nutrient detection, etc., and actuators such as lighting, watering, etc. This project will involve working with the MIB lab and MuLab to significantly expand the initial software for the first iteration of the system being built in 2023/24 academic year. It will be primarily based on the
Home Assistant platform.
Automated Agriculture Frontend
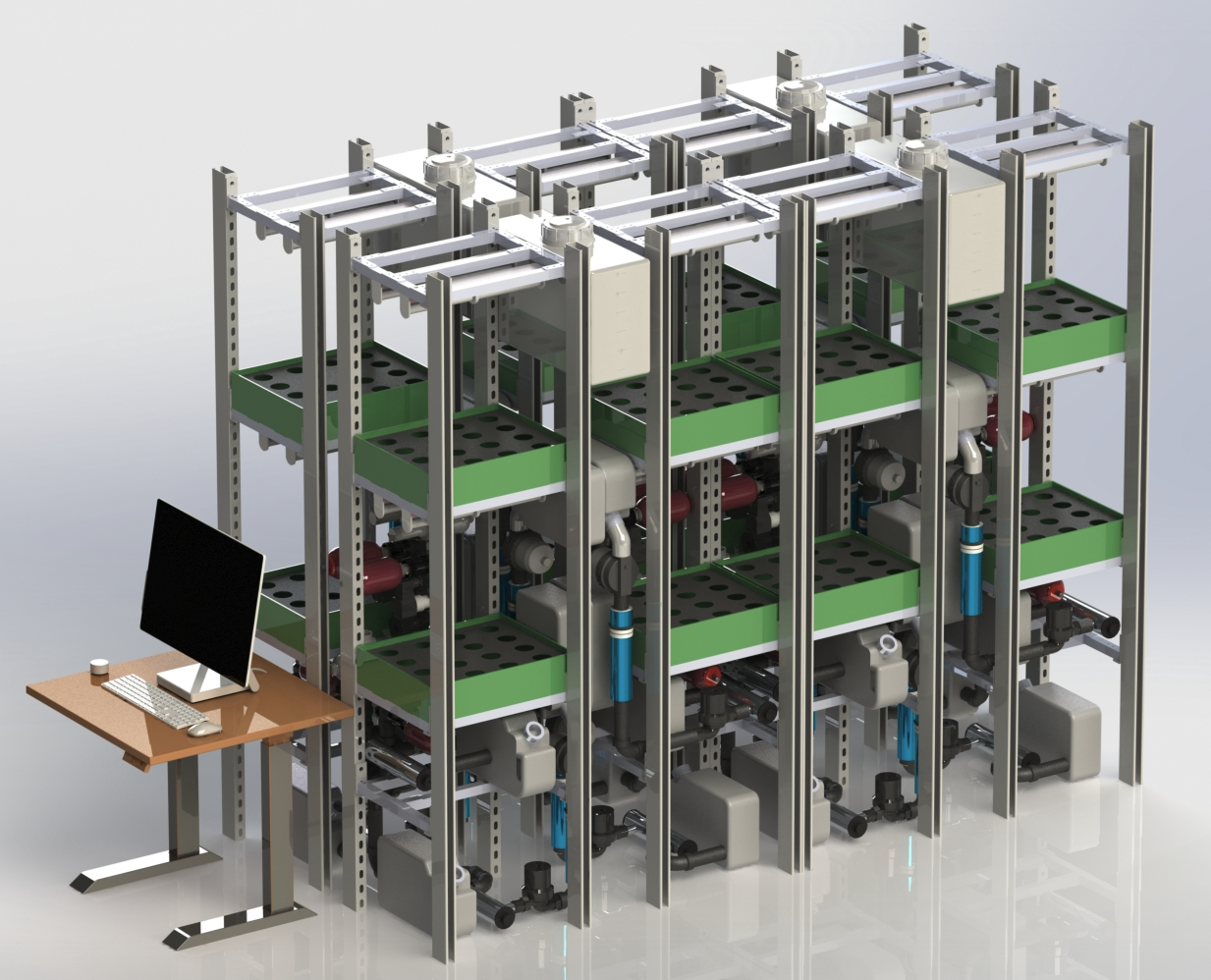
The MuLab and
Machine Intelligence & Biocomputing (MIB) Laboratory are building a lab-scale platform for the exploration of autonomous agriculture. This will include sensors such as video feeds, nutrient detection, etc., and actuators such as lighting, watering, etc. This project will involve working with the MIB lab and MuLab to build a frontend to both analyze the health and status of the plants, and control the system as a whole. The development will be focused on a web implementation that takes advantage of touch and dial-based inputs of the
Microsoft Surface Studio.
Research Ready Smart Watch
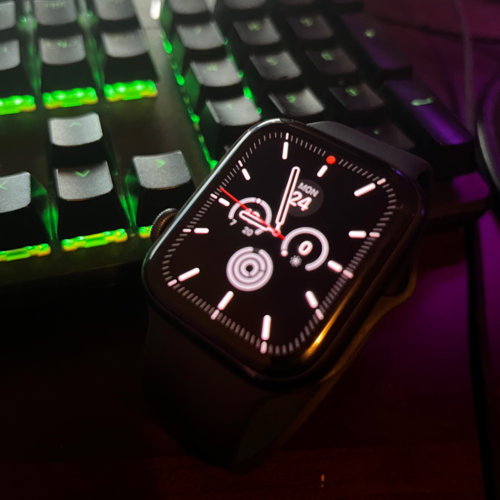
To conduct ongoing research in both continuous authentication and smart office analytics, this project aims to build a custom Android Wear application that will let us make use of all the sensors in modern smartwatches. Students located in Kingston will optionally have access to modern wearables in order to test the developed application, and the resulting prototype will contribute directly to research in both the MuLab and
CSRL research labs.
Campsite Scan
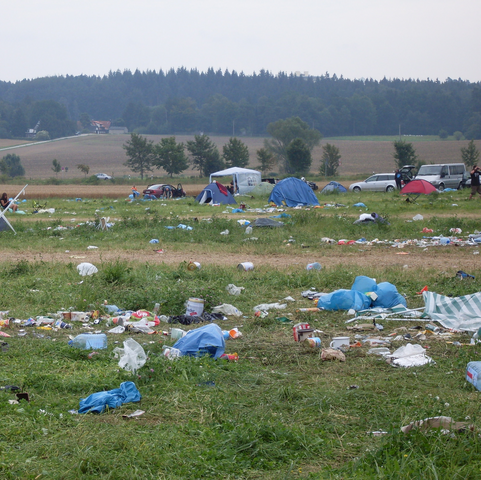
This research project aims to reduce the number of hazardous sites left, by requiring campers to
complete an automated checkout-safety scan. Following the leave-no-trace policy, the proposed
verification system will leverage computer vision techniques to ensure that fires are completely
put-out, and garbage is not left behind before campers are allowed to leave. The project will investigate
the use of pre-trained YOLO models for object detection to identify key items such as the fire pit,
wrappers, cans, and other belongings that may have been left behind. The system will also
further employ a model to detect if the identified firepit poses a risk (fire hazard vs fully
extinguished).
Computational Art Installation
This project will involve working with some number of members from the MuLab to visualize, artistically, some of the ongoing research. The final artefact will be an installation in Goodwin 627 (the MuLab), and this open-ended project is aimed at students who are in COCA and interested in the field of computational art.
(image generated using DALL-E 2)